Darwin’s theories in the artificial intelligence era
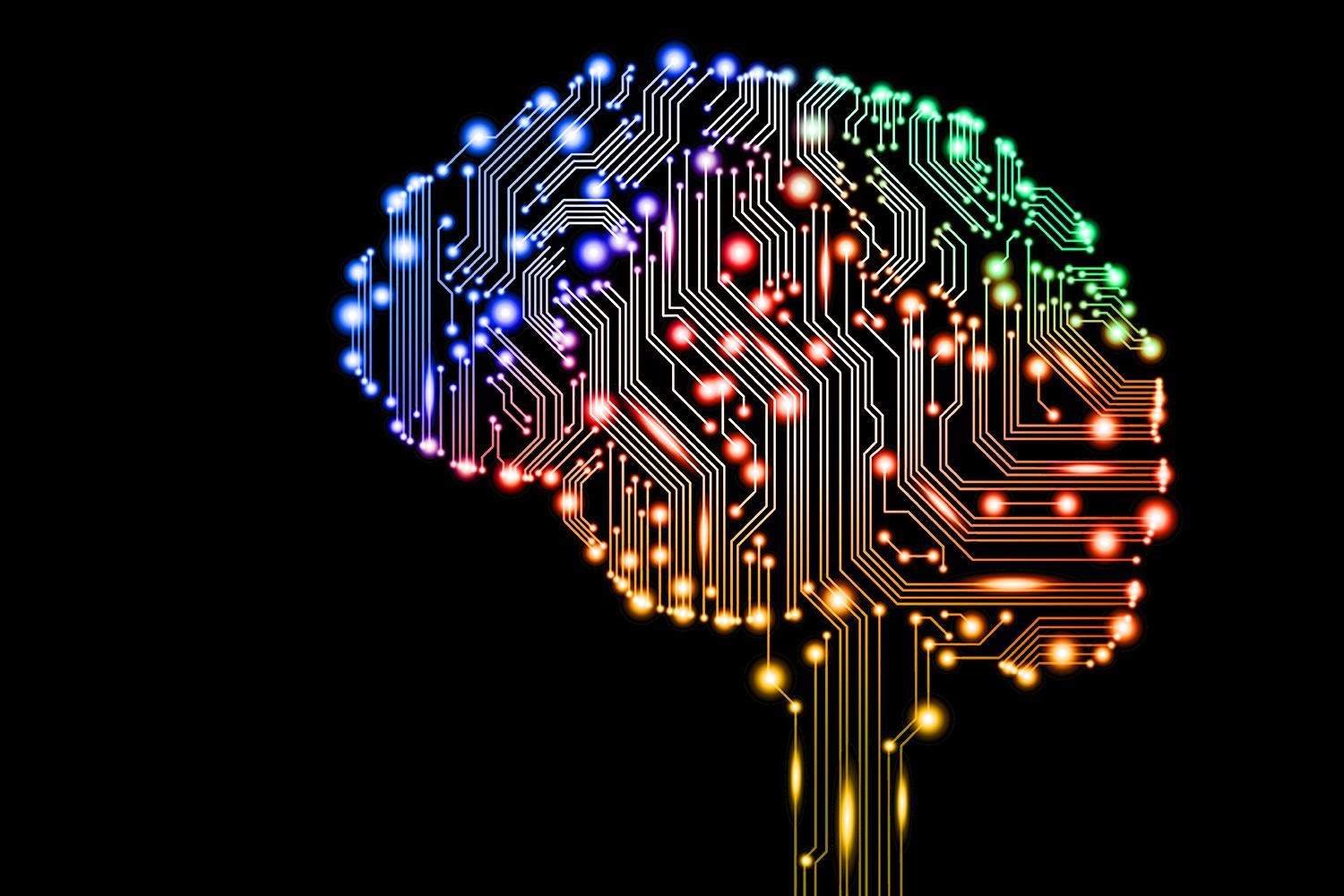
Artificial intelligence (AI) today is built to mimic nature, especially replicate the same decision-making process human beings have. AI’s brain-like development has surrounded the “neural networks” theme. This term, borrowed from neurobiology, describes machine thought as the movement of data through mathematical functions called neurons.
But now, computer scientists are considering putting AI through evolutionary processes to develop smarter and more efficient algorithms. This brings back Darwin’s concept of evolution which states that random changes in an organism’s genetic makeup will rather give an advantage or disadvantage in the wild. If the organism, then survives and reproduces that mutation will be passed along.
For algorithms this is known as neuroevolution. Whereas neural networks replicate the process of learning individual concepts, neuroevolution tries to recreate the process that built the parts of the brain. This concept has been known since the 1980’s but its gained attention nowadays thanks to Google Brain and non-profit organization OpenAI.
They each published unreviewed papers on the subject. Google explored the application of neuroevolution principles on image recognition while OpenAI used “worker” algorithms to teach a master algorithm the best way to accomplish a task.
Google generated 1,000 image-recognition algorithms that were train to recognize a specific set of images. Then 250 computers each chose two algorithms and tested their accuracy. The one with higher accuracy “lived” while the one that performed poorly was “killed.”
The survivor was then copied and slightly changed, just like human DNA randomly changes during reproduction. The mutation altered how the new algorithm interpreted data and was put back in the 1,000 batch to start the process over again. They found that neuroevolution could cultivate an algorithm with 94.6% accuracy and recorded similar results during each four repeats of the experiment.
By focusing on image-recognition Google tested neuroevolution’s capacity to tackle something the biological brain is great at and its ability to solve a modern problem.
Meanwhile, OpenAI used a purer form of evolution to undertake a different task. They used worker algorithms to train a master algorithm to accomplish an unknown task like playing a video game.
This technique teaches machines how to learn from specific information more efficiently rather than how to make decisions. Then the evolutionary algorithm is able to monitor how its workers are learning and learns to learn. Their experiment set 1,440 worker algorithms to the task of paying Atari. The ones that got the highest scores were copied and randomly mutated and went back in rotation.
Both experiments have their limitations but they’re an interesting example on how AI is evolving and how scientists continue to draw information from human beings biology to enhance and develop their technology.
Prepared by